- Product
AI Design
- Templates
- Solutions
- Product Managers & Product TeamsCreate mockups in seconds
- Designers & UX ProsDesign and iterate ideas in minutes
- MarketersDeliver for clients at speed
- Startup FoundersBring your startup MVP to life
- Consultants & AgenciesTurn ideas into interactive mockups
- DevelopersThe perfect launchpad for your project
- EnterpriseUizard for large businesses
- Blog
Uizard Research
Explore the research and technology that makes Uizard possible.
The scientific contributions listed on this page showcase some of the technology powering Uizard. The presented methods, experiments, and results are published for educational purposes only.
We share the science, but we keep some secrets. 😉
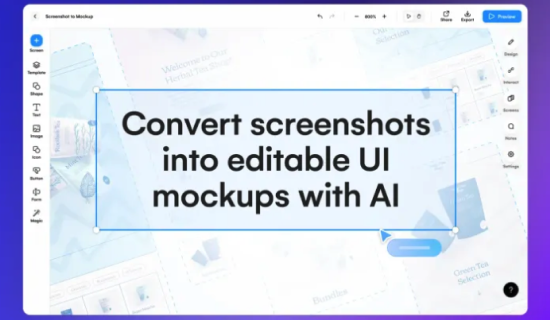
February 24 2023
Uizard Screenshot: Convert screenshots to editable mockups with deep neural networks
What comes to mind when you think of exceptional user experience? If you're a designer or a product owner, you probably have an idea of what makes great design; you might even have your own UI design playbook for crafting the perfect apps or websites every time you start a new project.
The truth is, refining your UI design to ensure you give your audience the best possible experience is a tricky task. Great UI design isn’t just about how your design looks, what colors you use, or the tone of voice you employ across your content...
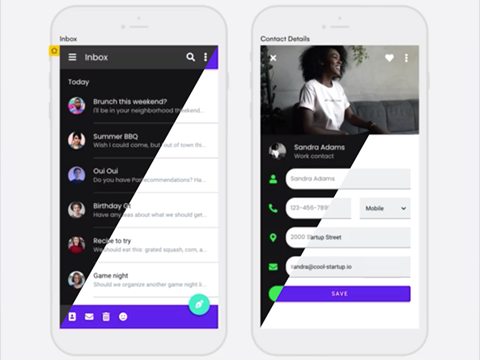
September 30 2021
How deep learning is transforming design: NLP and CV applications
If you have ever tried creating a user interface, you probably quickly realized that design is hard. Choosing the right colors, using fonts that match, making your layout balanced… And all of that while keeping your users’ needs in mind! Can we somehow reduce all of this complexity, allowing everyone to design, even if they don’t know about spacing rules or color contrast theory? Wouldn’t it be nice if software could help you with these?
This problem is not new. This is a long-standing problem that the Human Computer Interaction (HCI) community has been working towards for years...
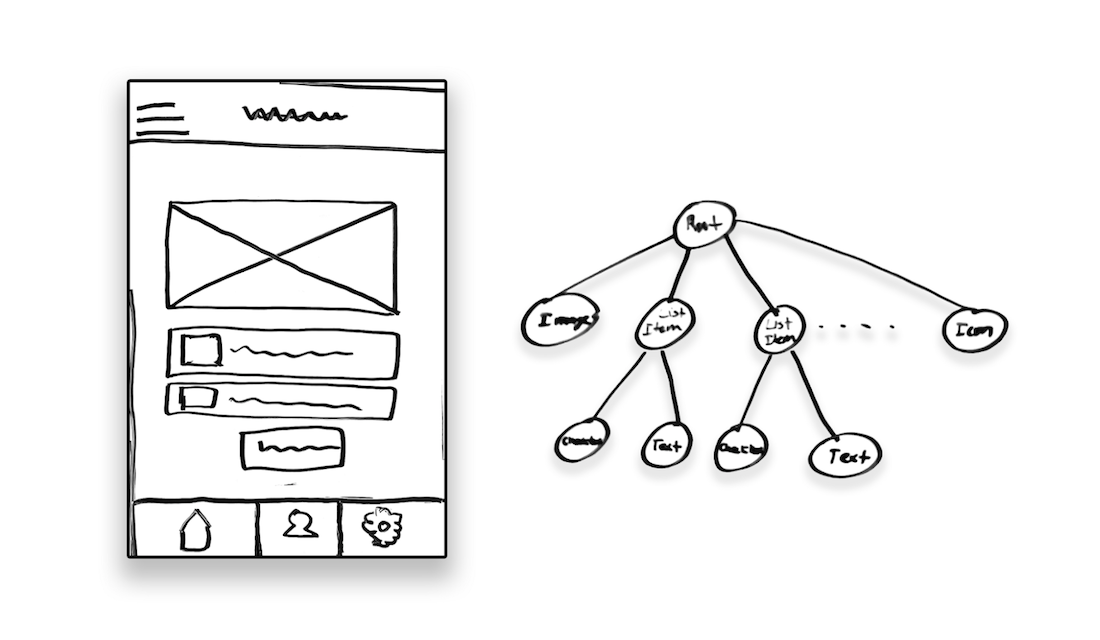
March 27 2021
Improving UI Layout Understanding with Hierarchical Positional Encodings
How can we modify transformers for UI-centric tasks? Layout Understanding is a sub-field of AI that enables machines to better process the semantics and information within layouts such as user interfaces (UIs), text documents, forms, presentation slides, graphic design compositions, etc.
Companies already invest extensive resources into their web/mobile applications' UI and user experience (UX), with Fast Company reporting that every $1 spent on UX can return between 2-100x on its investment.
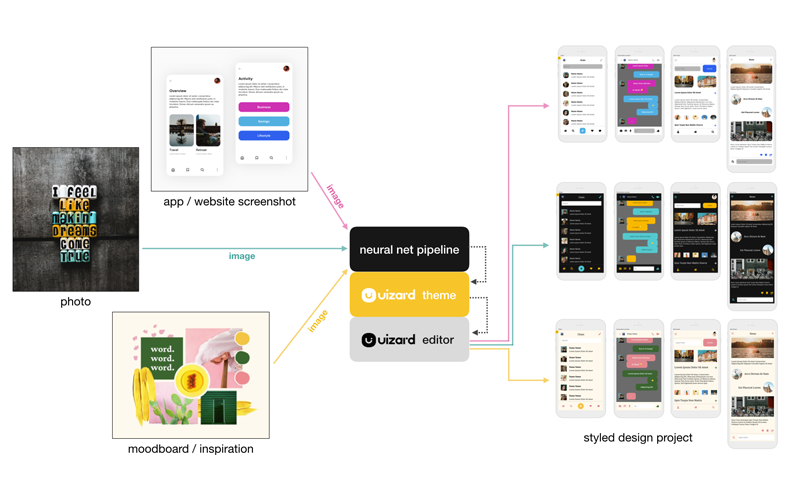
February 17 2021
Generating Design Systems using Deep Learning
How do you enable people to easily design beautiful mobile and web apps when they have no design background? Easy: you just throw some neural networks at the problem! Well, kinda...
You might have heard of Uizard already through our early machine learning research pix2code or our technology for turning hand-drawn sketches into interactive app prototypes. Today, I would like to talk about one of our new AI-driven features: automatic theme generation.
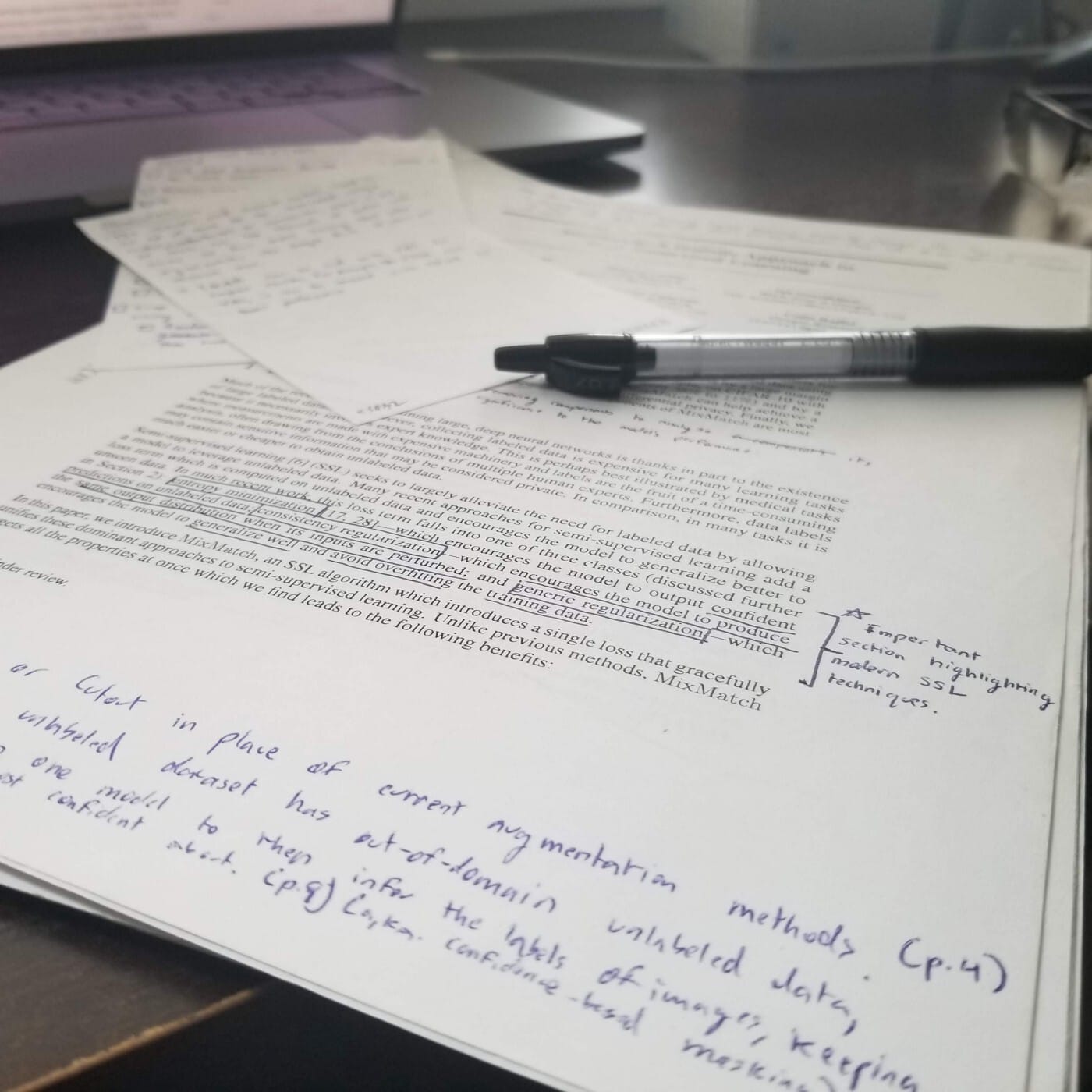
September 4 2020
From Research to Production with Deep Semi-Supervised Learning
Semi-Supervised Learning algorithms have shown great potential in training regimes when access to labeled data is scarce but access to unlabeled data is plentiful.
In this post, we discuss how Semi-supervisedlearning approaches can be useful for machine learning production environmentsand the lessons we've learned using them at Uizard.
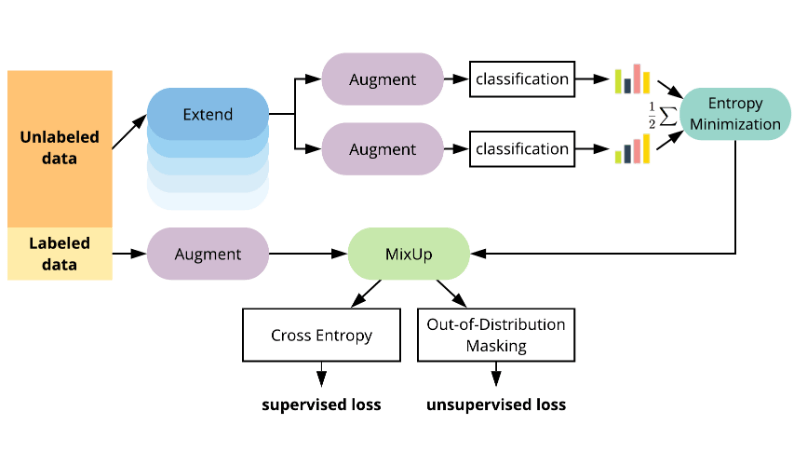
December 18 2019
RealMix: Towards Realistic Semi-Supervised Deep Learning Algorithms
Semi-Supervised Learning (SSL) algorithms have shown great potential in training regimes when access to labeled data is scarce but access to unlabeled data is plentiful.
However, our experiments illustrate several shortcomings that prior SSL algorithms suffer from. In particular, poor performance when unlabeled and labeled data distributions differ. To address these observations, we develop RealMix, which...
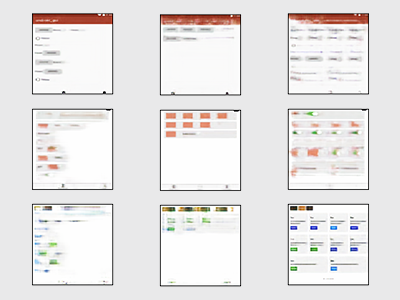
June 5 2018
Code2Pix: Deep Learning Compiler for Graphical User Interfaces
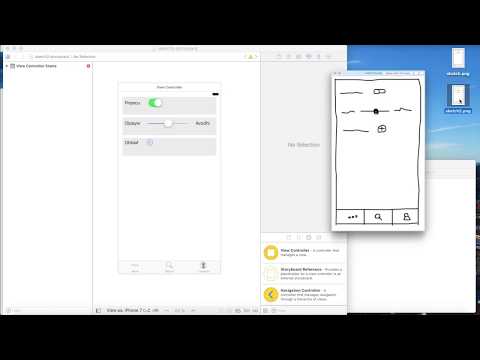
November 30 2017
Teaching Machines to Understand User Interfaces
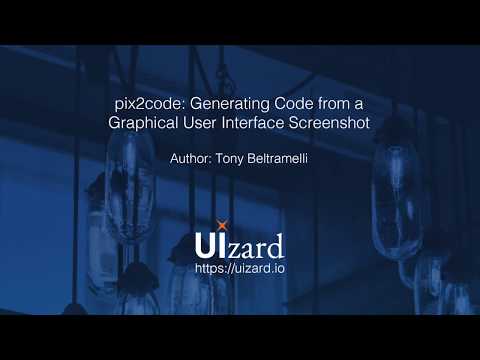
May 23 2017
pix2code: Generating Code from a Graphical User Interface Screenshot
Uizard
Templates
- UI Design Templates
- Mobile App Templates
- Website Templates
- Web App Templates
- Tablet App Templates
- UI Components Library
Wireframes
Solutions